Data Quality Monitoring: 3 Steps to Maximizing the Value of Your Data
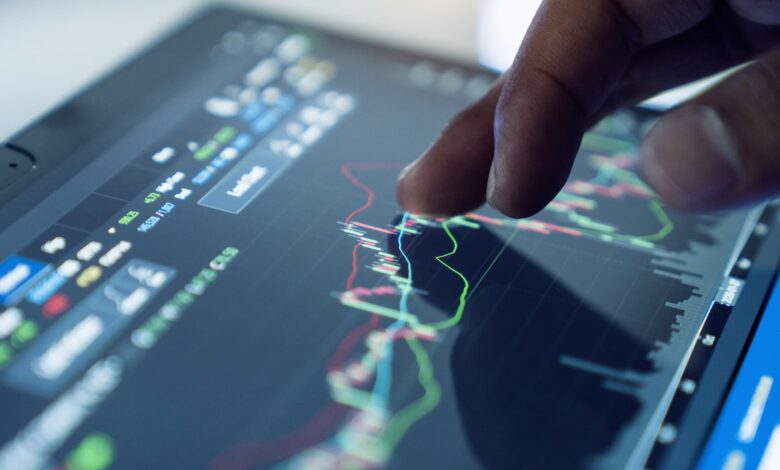
Nowadays, data is everywhere and businesses rely on it to gain insights, streamline processes, and make better decisions. As the complexity of data increases, ensuring its quality has become more important than ever before and should be a top priority.
What is data quality?
Data quality is a way of measuring how well a dataset is suited to meet the specific needs of an organization. While it may be easy to recognize good-quality data, it can be difficult to determine. That’s why relying on a single metric to measure data quality is not enough. Data quality dimensions are what capture the attributes that matter most to your organization.
While different experts have proposed different data quality dimensions and there is no standardization of their names or descriptions, almost all of them include some version of accuracy, completeness, consistency, timeliness, uniqueness, and validity. Before selecting which data quality dimensions are relevant to your company, you need to understand them. Typically, the following key dimensions are used:
Accuracy is the degree of closeness of data values to the actual values, often measured by comparing them with a known source of correct information. Inaccurate data cannot be used as a reliable source of information and impacts the organization’s business intelligence, budgeting, forecasting, and other critical activities.
Completeness is the degree to which all required records and data values are present with no missing information. Completeness does not measure accuracy or validity, but rather what information is missing.
Consistency is the degree to which data values of two sets of attributes within a record, within a data file, between data files, and within a record at different points in time comply with a rule. This dimension represents whether the same information stored and used at multiple instances matches. A lack of consistency can be observed when information stored in one place does not match relevant data stored elsewhere.
Reasonableness is the degree to which a data pattern meets expectations. It measures the degree to which data values have a reasonable or understandable data type and size.
Timeliness is the degree to which the period between the time of the creation of the real value and the time that the dataset is available is appropriate.
Uniqueness is the degree to which records occur only once in a data file and are not duplicated. Problems with uniqueness arise when the same data is stored in multiple locations.
Validity is the degree to which data values comply with pre-defined business rules such as the format, type, and range, such as zip codes and emails.
Maintaining high data quality is crucial for businesses.
It is essential for organizations to maintain data quality to make informed decisions, gain insights, and achieve their business objectives. Good data quality also enhances operational efficiency and supports effective decision-making processes.
However, with the shift towards storing data in data lakes or using data mesh architecture, data teams are faced with more data sources and complex pipelines. To deliver trustworthy data, it is essential to continuously monitor data quality and detect any problems before they impact your business.
3 Steps to implement data quality monitoring
If you’re looking to establish and maintain high standards of data quality, here are three key steps you should follow.
Firstly, assess your data quality needs and define clear objectives. Identify the specific data elements that are critical to your business operations and decision-making processes. Determine the quality requirements for each data element, including accuracy, completeness, consistency, and timeliness. By clearly defining your data quality objectives, you can focus your efforts on improving the most important aspects of your data.
Secondly, set up a data quality monitoring process that involves different stakeholders such as data owners, producers, engineering teams, and quality teams. The process should be based on tracking data quality KPIs to identify issues that should be addressed with the highest priority. The data quality KPIs and a list of tables affected by the data quality issues should be presented on data quality dashboards.
Finally, regularly monitor and improve your data to ensure ongoing data quality. This iterative process involves identifying issues, resolving them, and re-executing data quality monitoring.
If you’re looking for more information on data quality monitoring, our team at DQO has written an eBook that describes a proven process based on tracking data quality KPIs. The eBook is a great resource if you’re looking to improve the quality of your data and achieve a 100% data quality score.